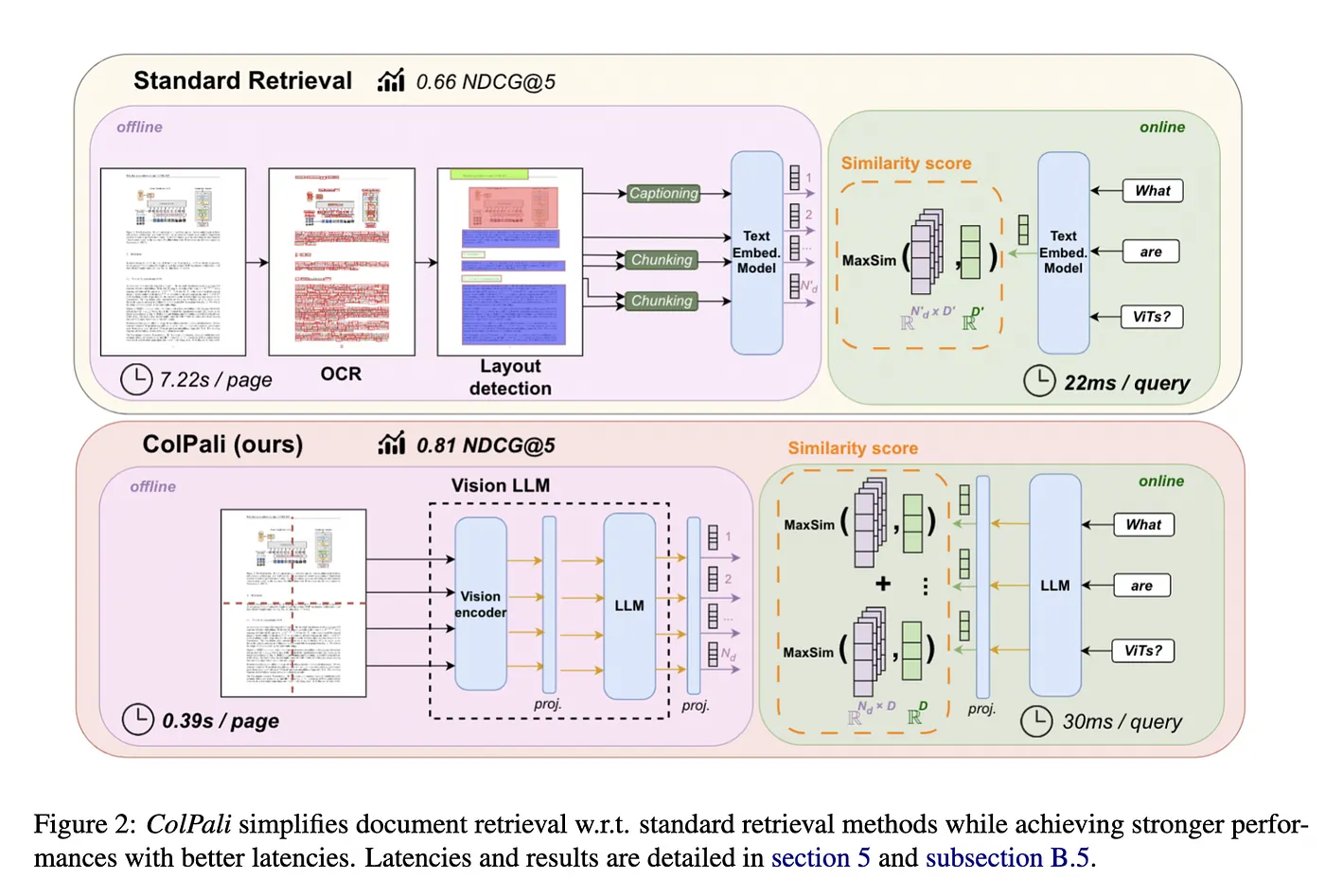
A new method for document retrieval, known as ColPali, has emerged as a significant development in efficiently handling visually rich documents, combining recent advances in Vision Language Models (VLMs). This innovative retrieval architecture, designed by Manuel Faysse, Hugues Sibille, Tony Wu, and colleagues from Illuin Technology and several academic institutions, addresses the limitations faced by existing systems that focus heavily on query-to-text matching. The paper details the introduction of the Visual Document Retrieval Benchmark (ViDoRe), which evaluates the performance of retrieval systems across multiple languages, domains, and document types.
Documents often include non-textual elements like tables, figures, and page layouts, which most retrieval models fail to exploit effectively. ColPali leverages these visual components to produce high-quality contextualized embeddings from document images. By combining this with a late interaction matching mechanism, ColPali is significantly faster and more accurate than current text-based systems. The research highlights the struggles of traditional methods, which rely on optical character recognition (OCR) and text chunking, and showcases how optimizing the data ingestion pipeline yields better results than improving text embedding models.
The ViDoRe benchmark, designed to test the retrieval system’s ability to understand both textual and visual elements, revealed significant shortcomings in existing models. ColPali, however, excelled, outperforming other retrieval systems in both speed and retrieval performance. It is particularly adept at retrieving complex documents like infographics, tables, and figures, making it a powerful tool for applications like Retrieval Augmented Generation (RAG), commonly used in industries such as healthcare and energy.
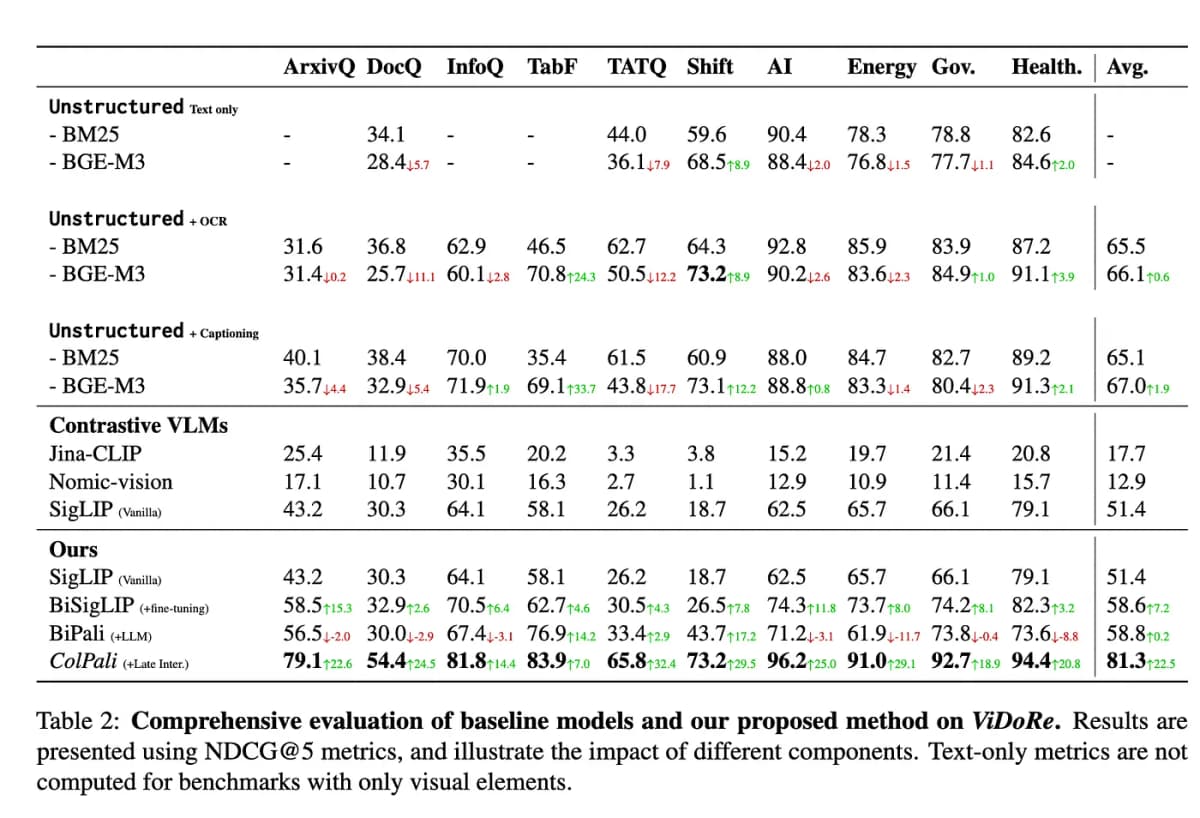
The researchers underline that document retrieval is a crucial component in industrial applications, particularly in search engines and complex information extraction systems. ColPali's ability to handle large corpora with minimal latency and its end-to-end trainability are likely to make it an essential tool for businesses that rely on accurate and fast document retrieval.
The research team has publicly released the ViDoRe benchmark and ColPali models, encouraging further developments and experimentation in document retrieval technology. Their contributions promise to reshape the landscape of document understanding by making better use of visual data, improving both the accuracy and efficiency of information retrieval systems.
Key Criticism:
Despite its innovative approach, the ColPali system raises concerns about overcomplicating document retrieval by heavily relying on visual data when text-based methods may suffice in many practical applications. The model's focus on Vision Language Models may lead to resource inefficiencies, especially in contexts where text dominates. Additionally, the paper's reliance on synthetic queries for training introduces potential biases, as these may not fully represent real-world user behavior.
ColPali's generalizability to languages outside of high-resource settings like English and French is also questionable, as the research lacks comprehensive evaluation on non-Latin scripts, which limits its applicability globally. Furthermore, while the model shows strong performance, its high computational demands, particularly for large-scale deployment, and ethical considerations around handling sensitive information are insufficiently addressed. Lastly, ColPali's reliance on pre-trained models limits its novelty, raising questions about the true extent of its contribution beyond combining existing technologies.