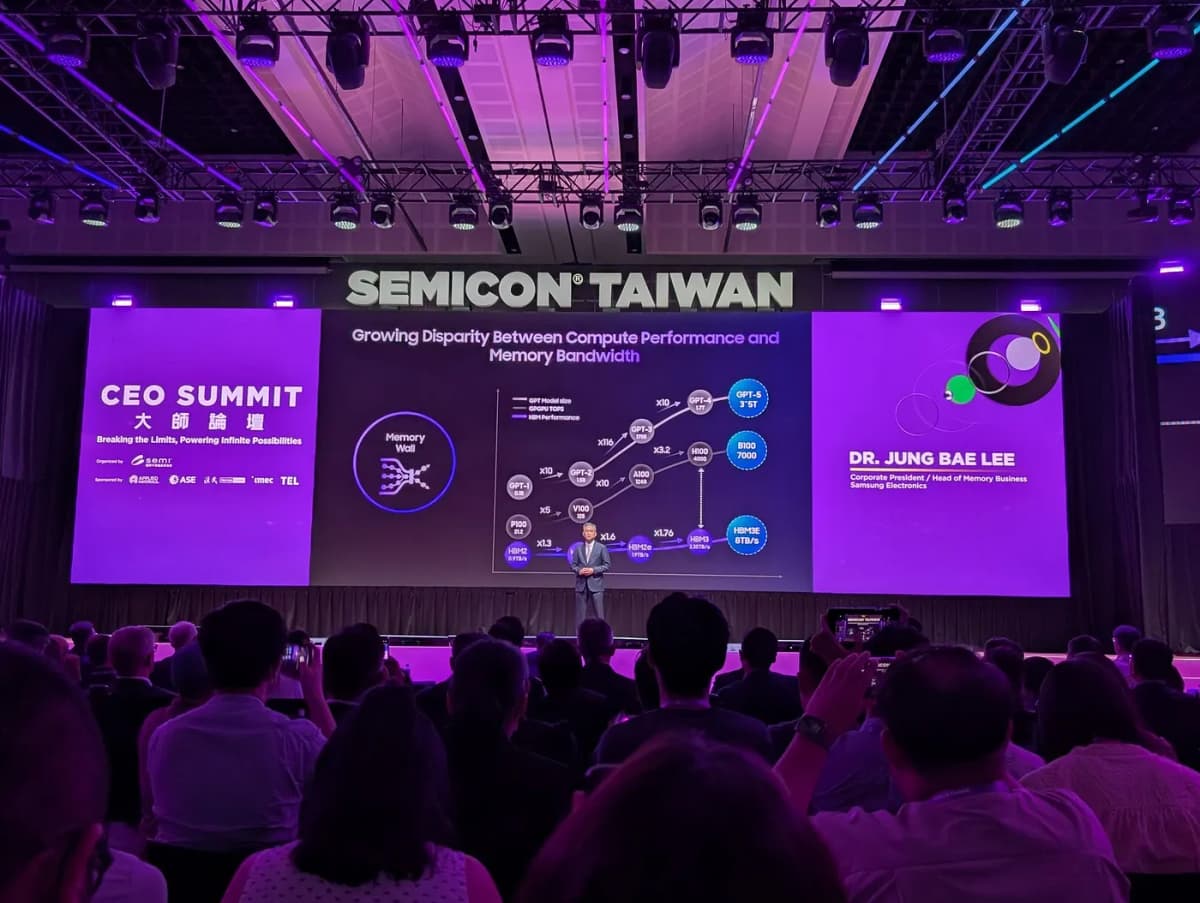
At the SEMICON Taiwan CEO Summit, Dr. Jung Bae Lee, Corporate President and Head of Memory Business at Samsung Electronics, stressed that the rapid growth of AI models is being constrained by a growing disparity between compute performance and memory bandwidth. While next-generation models like GPT-5 are expected to reach an unprecedented scale of 3-5 trillion parameters, the technical bottleneck of memory bandwidth is becoming a critical obstacle to realizing their full potential.
Dr. Lee’s slide, titled "Growing Disparity Between Compute Performance and Memory Bandwidth," underscored that although AI compute power has advanced significantly in recent years, memory systems are struggling to keep pace. This widening gap, often referred to as the "memory wall," threatens to diminish the returns of future AI advancements, despite the exponential increase in model size and complexity.
Memory Bandwidth: A Limiting Factor
At the core of the challenge is memory bandwidth, the rate at which data is transferred between memory and processors. For AI models like GPT-5, which require massive amounts of data to be processed in parallel, the efficiency of memory access is crucial. Dr. Lee explained that, while GPUs and other processing hardware have become incredibly powerful, they are often bottlenecked by the speed at which data can be supplied by memory systems.
Without matching advances in memory bandwidth, even the most powerful processors and the largest models will be left waiting for data, limiting their overall performance.
As models grow in size, the demand for faster memory increases. For example, GPT-5’s estimated 3-5 trillion parameters will require enormous computational power not only for training but also for inference—the process of making predictions based on the model. However, if memory cannot supply data at the rate required by these models, computational resources will be underutilized, leading to inefficiencies and delays in AI performance.
The Memory Wall and Its Implications
The "memory wall" challenge is especially critical for modern AI systems like GPT-5, where the gap between computational power and memory bandwidth has grown significantly. In these systems, although GPUs and other accelerators can perform a vast number of calculations per second, they are frequently bottlenecked by the speed at which data can be transferred between memory and processors. This results in idle times where processing units wait for data, limiting overall system efficiency.
As AI models scale—such as moving from GPT-4 to GPT-5 with trillions of parameters—the memory bottleneck becomes more pronounced. While scaling up the number of parameters can improve a model's ability to memorize and process data, it does not necessarily lead to proportional improvements in reasoning or generalization. In fact, diminishing returns may occur when larger models are hampered by memory constraints. For example, increasing model size requires exponentially more data movement, which can overwhelm current memory systems and reduce the potential for gains in model performance.
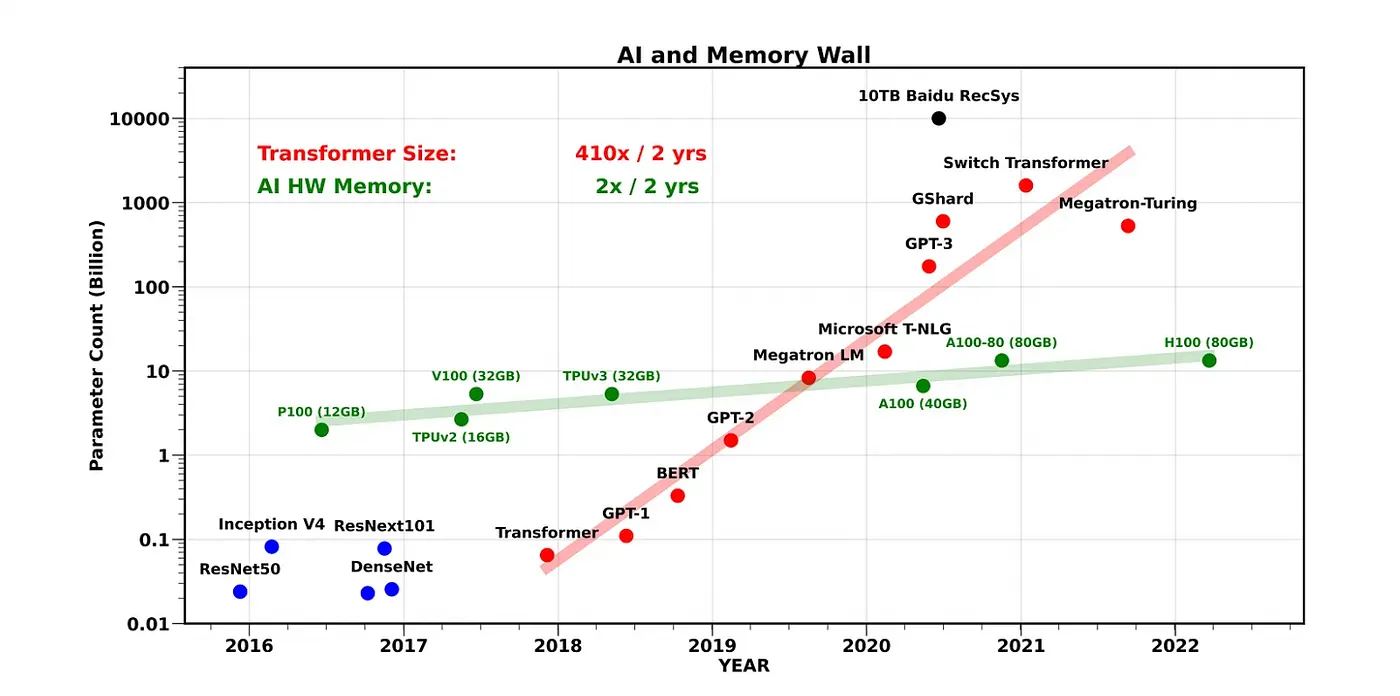
Image source: Amir Gholami, AI and Memory Wall
Innovations to Bridge the Gap
To address the growing disparity between compute and memory, several cutting-edge technologies are being explored. Dr. Lee highlighted some of the key solutions that could help overcome the memory bottleneck and enable more efficient AI models:
-
High-Bandwidth Memory (HBM3E): HBM is a specialized memory technology designed to offer significantly higher data transfer rates than traditional memory systems. The latest generation, HBM3E, is critical for supporting large AI models like GPT-5, as it provides the bandwidth needed to keep pace with compute-intensive tasks.
-
In-Memory Computing: One promising approach to mitigating the memory wall is in-memory computing, where some processing is done directly within the memory units themselves. This reduces the need to move large amounts of data between memory and processors, thereby improving overall efficiency.
-
Advanced Interconnect Technologies: Innovations like Compute Express Link (CXL) are improving data transfer speeds between compute resources and memory, helping to alleviate bottlenecks by providing faster, more efficient pathways for data movement.
-
Optimization Techniques: Beyond hardware solutions, AI researchers are also exploring sparsity techniques and model optimization to reduce the amount of data that needs to be processed at any given time. By focusing only on the most relevant information, models can operate more efficiently, even with limited memory bandwidth.
Looking Ahead: GPT-5 and Beyond
The technical challenges surrounding memory bandwidth underscore a broader issue in the development of next-generation AI models. While GPT-5, expected to be released around 2025, represents a significant leap in model size, Dr. Lee’s presentation highlighted that bigger models alone will not be sufficient to drive real progress in AI. Efficiency, optimization, and memory innovations will play just as important a role in the coming years.
The future of AI is not just about making models larger, but about making them smarter and more efficient. Dr. Leenoted that innovations in memory technology, like those being developed by Samsung, will be essential in enabling the next wave of AI advancements, ensuring that compute power can be fully utilized without being held back by memory limitations.
For companies and researchers pushing the boundaries of AI, overcoming the memory bottleneck will be key to unlocking the full capabilities of models like GPT-5 and future AI systems. Without it, the promise of larger models may fall short, with only marginal improvements in real-world applications.